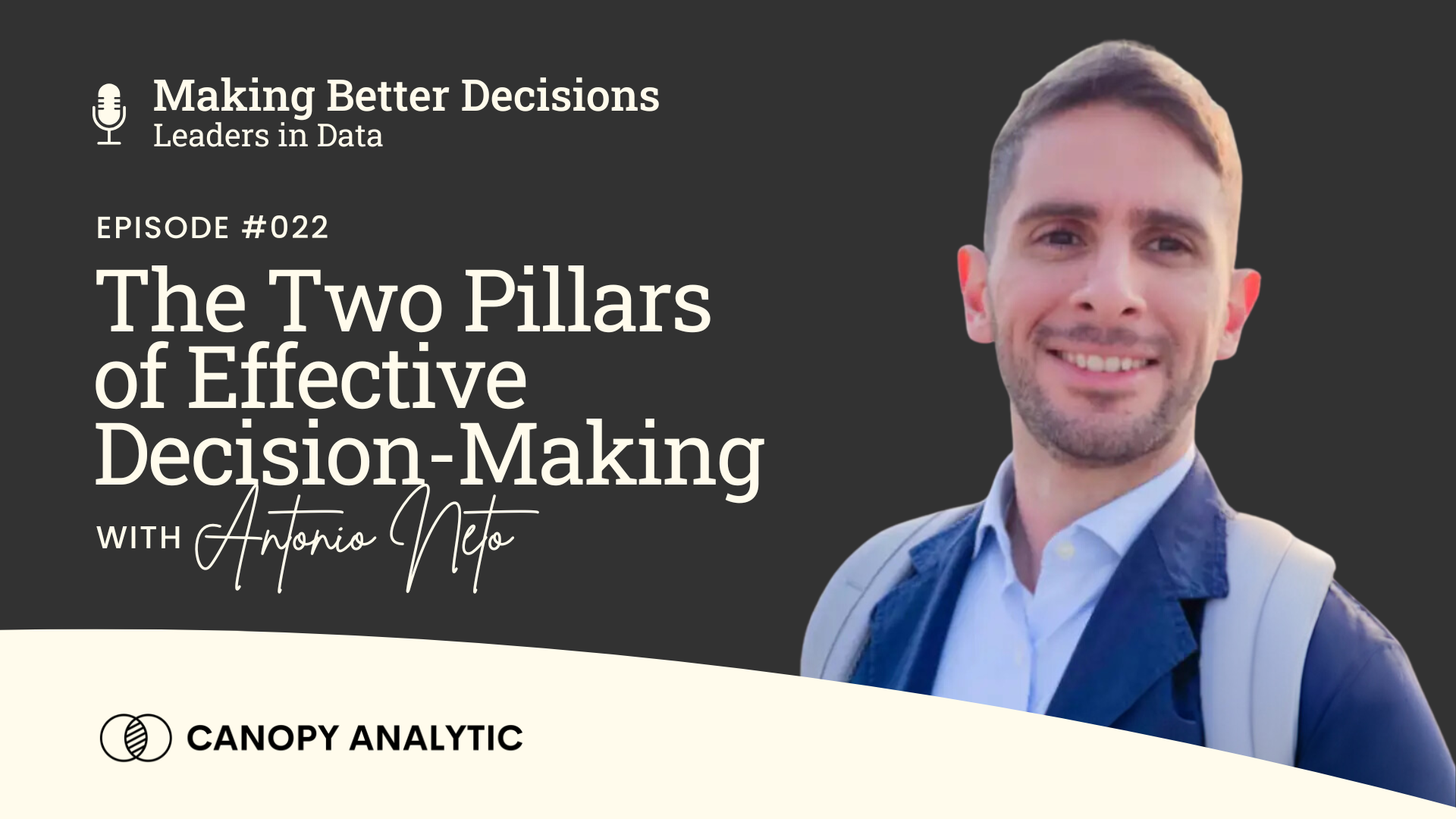
Data Quality and Problem Statements: The Two Pillars of Effective Decision-Making with Antonio Neto
This is Making Better Decisions. I’m your host, Ryan Sullivan. Decisions are where rubber meets the road for organizations. Each week, we’ll be learning from people who are on the front lines of turning raw data into better outcomes for their organizations. This show is sponsored by Canopy Analytic, helping companies make better decisions using data.
Ryan: welcome everybody to another episode of the making better decisions podcast Today’s guest has founded multiple companies and has been involved heavily in the startup space including a company from Y Combinator 2021 with multiple successful exits He’s held Positions as head of product, CFO and CEO, has a master’s in FinTech from NYU, has focused on giving back to the entrepreneurial community through mentorship.
Please welcome head of data and financial products at Capital, Antonio Netto.
Antonio: Hey, thank you. Thank you for having
Ryan: [00:01:00] I’m super excited to have you here. So I’d like to start with our springboard question. What is one thing you wish more people knew about using data to make better decisions? Hmm. Hmm.
Antonio: thing for me is, uh, understand, first of all, know how to ask questions for the data, because, uh, sometimes people wanna, want to have some information, but they don’t know how to make questions, how to create a, uh, problem statement, right? Uh, and when you don’t have that, uh, understanding, probably, uh, your answer is not And then you blame the data, say, Hey, the data does not work.
It’s hard to take a good decision. So on so forth, but in reality, the point is what do you want to know? Right. Uh, have that kind of, um, Understanding, uh, it’s [00:02:00] essential, it’s crucial for people to start to, uh, play with data and use data to take, uh, better decisions. That’s, uh, the number one thing. And, uh, the number two thing, uh, it’s a little follow up.
It’s, uh, Uh, quality of the data. If your data is not that good, probably, even though if you know how to, uh, create the problem statement, uh, your answer will be poor. And that, uh, for me is the two, uh, pillars, uh, to really master in, uh, make decisions based on data.
Ryan: Yeah. I think that that’s a common theme with a lot of the folks that I’ve spoken to, this kind of idea of learning how to craft the problem statement. And sometimes the focus is on, you know, okay, well, Are we tying this to a business objective? Sometimes it’s more about having an understanding of how the business’ data [00:03:00] mirror its processes, so kind of where to go to find what you need. What is some advice you could give to someone who’s maybe aware of this problem, but wants to improve their ability to ask better questions?
Antonio: Perfect. Well, better questions. Uh, the fundamental is. Understand your doubt, uh, write down, uh, pick a piece of paper and literally, uh, write what I want, what I want to understand about it and, uh, write and read and see, okay. make sense, uh, what I’m asking for. Uh, if somebody else asked it to me, uh, I will be able, uh, to answer, uh, that question or not.
That critical think, it’s a, it’s essential, uh, looks like a simple exercise, but, uh, it’s not that simple because it make people think about what they really are. Uh, want to know, right? [00:04:00] Uh, if, uh, what they want to know really, uh, match with the goals, uh, that he or she, uh, might have, uh, it’s often, uh, people say, Hey, I want to understand, uh, X and Y and Z.
And when I ask, but why you need to understand? Oh, because I want to see if the consumer will go up or down in the extensive direction. Okay, but what you’re asking for won’t tell you that answer and even won’t give any clue for
Ryan: Hmm.
Antonio: Let’s understand What you really want to learn. Ask for yourself. What do you want to learn?
Oh, I want to learn that movement, the verbals that eventually, uh, have a impact, uh, in that point that I’m studying, that it’s important for the company, et cetera. Uh, I want to learn If you know that, you can [00:05:00] make the right question. That’s the, uh, that’s the point for me, the simple exercise to, uh, write, uh, what you want and ask for yourself what I want to learn.
That’s always is the first question that I ask for people when they say, Hey, I want that information. I really believe that I need some insights because, you know, we need to improve the market and we need to improve, uh, uh, some, some needles or some, some KPIs for the companies. Perfect. Uh, you are right, but what do you want to learn with that information?
How can help you in that situation? Uh, if you don’t know what you want to learn, probably, uh, You’d want to create a good, uh, questions for the data. And often what will happen will be the data science team will try to figure out what we really, uh, want to learn. And that’s painful because, uh, often what happened is, [00:06:00] uh, based on your question, the data team will start to, uh, dig in some, uh, in some investigation, make some experimentation and show something for you and, uh, you say, Oh, It’s not that thing that I want to learn, or it doesn’t matter for me.
And say, okay, but you asked for that, right? So, uh, what really we can change in that experimentation, that investigation to help you? And that point, the person will, uh, explain to you what really matters, or what they, she, uh, or he want, uh, to learn. That is the, uh, the main thing.
Ryan: Yeah. I think that, you know, obviously the name is in the podcast where I think the rubber hits the road with analytics and it’s all around business decisions. And I think you’re, You’re kind of driving at that a little bit, right? Like what, okay. What’s, what’s the business call we’re [00:07:00] trying to make here.
Are we trying to sell more of something? Do we want to find out if people like something, do we want to find out how many people we need, whatever it is, if I can drive it back to that business question, number one, you know, even if we just assume that that person is going to kind of go get it on their own, going through the exercise of figuring out like, what is the actual decision?
Like, once you have this analysis, what are you saying? Yes or no to. It also helps, like you mentioned, right? If you have a data science team or if you have a centralized reporting function, or even you just have like, you know, wizard analyst, they frequently know a lot of data that’s out there. So maybe you’re coming in and you’re saying, oh, okay, well, we want to see how long people lingered on.
The landing page that we had for this new product. Okay, awesome. So you go and you say, we’re trying to figure out whether people like the product that analysts could come back and say, Oh, well, maybe there’s some other information in another part of the business that you didn’t know about. Like we have [00:08:00] information on X, Y, or Z.
That shows that they may like the product. So again, it kind of provides if you have that problem statement, it puts everything into this unique language where then everybody on the team can get aligned with it. Now I couldn’t help, And my example, they’re talking about products because I know that that’s something that you have a lot of experience with.
So when we’re talking about product development, product management, product market fit, talk to us a little bit about how data plays a role in managing and developing products.
Antonio: Well, for me it’s, um, it’s a, I love that topic, and, uh, uh, it’s essential. It’s a crucial, uh, because, uh, currently we have some detach and between data teams and, uh, product teams. And, uh, really for me, it’s, uh, not the best way to do things because, uh, data is a crucial part to understand, to develop, [00:09:00] uh, any kind of product.
Uh, what I meant to say is. The data will tell you a bunch of stories about the user experience, how the user, uh, uh, are using your product, how, uh, and where, uh, they have a problems to use your pro your products, and you can very fast, uh, understanding and, uh, change, uh, your product. Uh, what I’m seeing currently, especially in startup world, People don’t, uh, they want to be data driving companies, but most of people don’t know how to build that infrastructure or even how to use data, right?
How to track the user behavior, how to analyze that user behavior in order to improve the product. Or even [00:10:00] instruments in data space, uh, to take the decisions. What I’m going to say is a lot Often, uh, a lot of people say, hey, I should, uh, do X on Y things with me, uh, for that product, right? Uh, should I change that, uh, landing page?
Should I, uh, change, uh, some part of the product? Uh, and also my question is, let the data speak. What I mean is, Uh, let’s, let’s do a A B testing, for example, let’s see how, uh, the data will tell the story for us, take the decisions based on that. Often I have some pushbacks and people say, Oh, but it takes time.
Uh, yes, it’s a, sometimes it will take time to create A B testing. You should think, uh, it would take a month, a month and a half, then I will take a really long time. Data driven decisions. Instead of, uh, I think the decision 15 days that that 15 [00:11:00] days will become three months, a year till you find, in fact, the product to market fit, right?
That’s, uh, that’s, uh, another point or even when, uh, people don’t track, uh, data from the users, right? What I’m going to say is imagine that you have an app and you have different screens that, uh, people has, uh, Especially the product people that don’t have a lot of familiarity or, uh, don’t use data a lot, they don’t know how to create that trace.
Uh, and it is absolutely a marvel when you use, for example, one startup that I work with, uh, we have a, Terrible drop off in one part of the, um, of the flow of the onboard. Uh, when you look the screen by screen, okay, but why, right? Uh, but what’s weird, the flow and, uh, when say, okay, let’s do a B test, keep, uh, that, uh, flow as if, and let’s change [00:12:00] that screen to the beginning of the flow.
And see what happens. It’s just, we reduced the drop off in 80%. Just change, because that’s the flow that the user, uh, understand. Yeah. We just know that after a test and the track the data and they said, Oh, that’s the point that you have drop off. So that’s the point that you need. You should investigate.
So data is. And, uh, the most important thing for me in product data part is you start with the data since the beginning. Uh, what I want to say is, uh, I bootstrap in different companies. I start companies from zero. And, uh, of course, sometimes you need, uh, to speed up things, but it’s important that you, since the beginning, um, Understand that it’s important to track, important to create the commentations because you create that culture inside of the company [00:13:00] and everybody that you, uh, you, uh, arrive from them with the new employees, you understand that’s the culture of the company.
So that’s the way to build the products, uh, here. And your life will be much better and find the product marked fit, uh, will be less gamble and more scientific. That’s the importance of the data. Uh, let’s use everything that you have in currently. Ransom experimentation is not that expensive, uh, is not that complex.
So, uh, with JGPT and, and Trump and other things, a lot, a lot, uh, a bunch of analysis that cost a lot of time to do in the past, now you can do, any person can do it. Uh, even if you don’t have, uh, a, a huge, um, track record or understanding about data. Currently, you can use that kind of a solution. So my call is use data since the beginning.
[00:14:00] Uh, understand that you have to track data and understand that you have to analyze user’s behavior since you create your first draft, your first plan of your company, your first product already should be shipping in that direction. Otherwise, It’ll be difficult because if you don’t create that culture inside of the company, uh, it’s difficult to change, uh, after one, two years, you say, Oh, now I have to, to take care of the data.
And you’ll have whole developed team that sometimes don’t have that culture because you did not start with that and you have to change mentality. And that’s the difficult part. That’s it.
Ryan: everywhere, but I think one of the big things from the product world is this idea of fail fast and like try out a lot of things, but then if they’re not working, throw them away and start focusing on the things that do. And [00:15:00] I think that that, I’ve seen a lot of people say, okay, great.
Well, I’m going to try a lot of things, but the other half of that is we have to have a good idea of what’s working and what’s not working. And ideally we want to have that picture as quickly as possible. And you talk about how, like, you know, if I have data to know, You know, in your page example, that the, the, the drop off was 80%.
And then you fix it, you know, the, the drop off was very high and then you fixed it by 80%. If you didn’t have the information to know that you just kind of be like, well, which one do we like better? Which one looks better? Um, you know, so I, I really do think that having accurate information about what’s working and what’s not working as kind of a necessary component of that fail fast mentality.
One of the other things that I think is really. interesting about this space is I’m by no means heavily educated or experienced in it, but I love a lot of the concepts of design [00:16:00] thinking. And when I have invested time into learning a bit more about design, you start to realize like, Oh, this is like a framework for thinking about every part of the world.
Right. It doesn’t just have to be products. It’s really just thinking about how do we make things? How do people use things? How do people think about things and then trying to map into that? So I think a lot about analytics and how applicable that design thinking is in analytics, right? If I just, you know, throw a bunch of information up.
Are people going to make the right decisions? How do they model that report in their head? How can I create for them? How can I present information in a way that it’s taken in an unbiased way and it’s consumed quickly and accurately. So I think that it’s one of those places where there’s just this massive opportunity.
Cause like you said, even though the skill sets of like going out and making a great product or then like building data engineering and reporting, those are very different things. Skill sets. But I think a lot of the governing thinking around like [00:17:00] how to design for people, how are people going to use or consume this information or product is actually really similar.
Antonio: Absolutely. Similar. Absolutely. Similar. Uh, you are absolutely right. For example, what I teach always for my team is, uh, literally do design. Think about what you want to do, what reports you want to create, uh, how, uh, You can visualize, I’m very visual person, so, and I love to, to work with that. Uh, okay. How you can build in visual way and talk, uh, with your consumer.
Let me, let me use example that you are using in, uh, inside of the company. That will be a report, for example, create. Discuss with them, see, uh, what is the pain that the, uh, that internal user tried to, uh, solve, and, uh, create your design, create literally, okay, which part will go in [00:18:00] that direction, how the person will see, uh, that report, and so on and so forth.
Design thinking help, help on the analytics part. Help as well. Uh, even when you think about build a data infrastructure, you need to use, you need to design think, uh, you need to. Okay, what, what that infrastructure will look like, how that data will flow, right? Uh, consume here, comes from him, you consume that part, how all things, uh, will, uh, be orchestrated together.
And I will, uh, go a little further. When you think about build products based on data for AI, That’s doing that. It’s become crucial because see that flow, understand that flow. It’s each day more crucial and make you, gives you some kind of a differentiation, even though in the market, because it’s not a [00:19:00] common set of skills, unfortunately, uh, in the data space.
That’s very technical to, uh, to have, uh, designing thinking before you start, uh, build anything.
Ryan: Yeah. One of, and I know that this is like a lifelong pursuit to really get your hands around design thinking, but you know, for where I’m at, one of the big takeaways that I’ve had is whenever I design something, I’m designing it for someone. And it’s that for someone that is really critical, right? Like what’s my audience, how do they think about it?
How do they use it? What are, you know, different things that I can learn about that person? Are there different types of people in the audience? And so I think about that a lot, as far as like, you mentioned it with data infrastructure, right? If. If I’m starting out building some big project and I’m not ultimately thinking about who are all of the types of people who are going to consume this information, how are they going [00:20:00] to consume this information?
What business decisions are they making? How is it even possible to build the right thing?
Antonio: And my, in my opinion, it’s impossible, but, but we see a bunch of people doing that because what happened is, uh, it’s one question not to have empathy for the, for whom you are building, for whom you are, uh, work with and, uh, often people don’t. tend to build the products based on, uh, when I say products, broadly speaking, data products, uh, products per se, uh, based on their own experience, their own, uh, okay, uh, I will build that because I’m feel that that part is painful.
Uh, it’s difficult for me. And, uh, in the reality, sometimes it’s match with the, what people, uh, the, your consumer target audience wants, but, uh, it’s a by chance. Not because you design for, [00:21:00] that’s the big challenge. Especially, it’s very good what you said, because especially in the entrepreneur world, uh, and I work as a mentor for different startups as well, and, uh, a lot of the entrepreneurs start with big ideas that solve their own problems.
Yeah, the owner pain say, okay, it could be interesting, but, uh, you, but you don’t need to build it for you. If you want to build it for you, you can build in your own laptop and that’s fine. But if you are building for somebody else, you need to talk. You need to understand if it’s a really a pain for somebody else.
If you have a market there, right? Uh, doesn’t matter if it’s important and, uh, for your target, chance is not. Uh, I, all the time in my experience in developing product and data, people say, Hey, uh, what do you think about that? And I said, okay, I’m terrible thinking in that sense. I have to
Ryan: Yeah. [00:22:00] Yeah.
Antonio: test with, uh, because eventually I’m not the target audience. And then often products that I develop, I’m not a target audience. So, uh, I have to understand what the target audience really needs. If that, uh, product is good or not. I had one time I was doing one product and they say, okay, uh, for me, it’s better in that way and, uh, the content and design team want to go the other direction and say, okay, let’s test.
If you don’t have agreement, let’s test. Let’s create a test. Uh, I was wrong. I was wrong because I was looking with my, uh, glass and, uh, I just saw one perspective of the problem and the audience shows no, the, uh, the other part of the team was right. Okay, let’s do, uh, all the time. You have to ask for yourself.
I saw, uh, uh, uh, A few years ago, I, [00:23:00] uh, I saw an interview, if I’m not wrong, it was the CPO at FIBAE, and she also, also from time, she worked at a CPO to Nike. I don’t remember exactly her name, unfortunately, but, uh, she said that, uh, she has a bunch of glasses. Uh, Sunglass in his, uh, in her house. And, uh, when people ask, Why do you have that bunch of, uh, sunglass?
And she said, because I need to put it to see the world with different colors, different visions. Because people is different. It’s remind me. That I have to do that, that part’s a crucial. And we have, when you’re looking for a startup to have different, uh, problems to fail, right? Sometimes startups, uh, don’t pivot fast.
Startups don’t, uh, find a product market fit or don’t have money, uh, run out of money, but a main problem that they have exactly that when they chase [00:24:00] the product market fit, they still fail. Spend too much time in the wrong vision, in their own vision of the product instead of the market. And what happens often is, when the entrepreneur realizes that, oh my gosh, I should go to the left instead of the right, it’s too late.
They just don’t have more money. They run out of money. And that, that’s a big problem.
Ryan: Yeah. So that’s actually a perfect place To pivot into like another area of your experience that I think will be really interesting to the listeners. So having been a part of a couple of startups, I know that there are a lot of different considerations there than if you’re at a larger, more established company, I think one of the big ones that you talked about is, is time and cashflow and cash pile and kind of all of these things, lots of times when we talk about.
You know, what’s the [00:25:00] best, you know, most right way to do things with data. It can be really easy to go straight to this like super enterprise, large, you know, whatever. And for a startup, that’s not the case. And so I think sometimes there’s, there’s this idea of like, either I have to shoot the moon and do everything the way that a fortune 10 company would do it, or I’m not ready for data.
And, you know, I obviously know that there are many, many steps of data maturity, wherever your company is. You can find a level of data maturity to match that. But as someone who’s been to that rodeo a couple of times, what’s some advice that you can give about picking the right investments? When is it worthwhile to invest in certain pieces of data and what things make sense earlier on?
What’s, what’s one of the best ways to think about doing data at a smaller company?
Antonio: That’s perfect. And you’re absolutely right. You have a bunch of tools that’s quite expensive that, uh, it’s become, uh, inaccessible for some startups. [00:26:00] Absolutely. Uh, absolutely right on that. But simple things, uh, it’s important and, uh, make whole difference. For example, uh, data quality. When I say data quality is how you build your, your software, your product, how you start that information, the documentation that you create as a For that, uh, uh, for that, uh, documentation, it’s often in your startup is, uh, it will be fun what I say, but it’s, it’s happened a lot.
Uh, they just override the data and they
Ryan: I didn’t hear that. No, never delete.
Antonio: Literally. They just overwrite the data. When you say, okay, I want to set up a device one time, I say, let me see, let’s try to, uh, look, look inside of the data that you have. You can do some study, some analysis. I can help on that and, uh, end up doing what’s wrong with the data because everything’s overwrited.[00:27:00]
They just lose six months of data because everything that they have, they just override helps just for 15 days. So, uh, it’s not that expensive. You create the documentation. It’s not expensive. You build thinking about, uh, that data that you have will be important at some point to, uh, To take decisions. So, uh, what are, what, what is the cost for create the right documentation? Okay? When one hour more for the developers will be that cost, but it’s, it won’t, uh, break, uh, the bank, right? You can afford, uh, create the write documentations. So, um. Look for some solutions in the market that will help you to track the user data. Sometimes, okay, you cannot afford the most, uh, fancy, [00:28:00] software tool that will, uh, help you to create the most, uh, fancy dashboard, but your team can track the data and you can analyze that data, especially today for me, we have, since we have, uh, new tools and are very affordable is not more excuse, uh, to don’t, uh, preservate that data, uh, create the right documentation.
That’s quite cheap for you, for you have, and, uh, understand that the data will be useful. Eventually we don’t have the capacity, financial capacity and technical capacity right now, but for sure. The most, um, junior developer, he knows how to store that data in a proper manner, right? He or she could not know how to do with that.
I mean, what information, uh, we can extract, keep that data.
Ryan: Yeah, that is, that is just like, so whenever I talk about this stuff, I [00:29:00] obviously, as a consultant and as the podcast host and all that stuff, I spend a lot of time talking to people about like, okay, here’s a data maturity curve and where’s your company curve and let’s match those up and get you where you want to be.
A lot of that conversation is always kind of so dominated by like, what is the right thing to do? tool to use, like how much backend infrastructure and am I building database, all this stuff, that advice just cuts right through to the heart of it. Right. Store everything. Cause then you have it. And if you want to use a lightweight tool or you want to use a heavyweight tool or whatever, you have it.
But keeping all of that data is just phenomenal advice. And I, at least I don’t talk about it enough. That’s a really, really good point.
Antonio: you have, you, you might keep because, uh, Otherwise, it’s just gone and, uh, you can recover, you can
Ryan: And storage is cheap.
Antonio: Exactly, exactly. Uh, of course you have sometimes, uh, have some, uh, big cultures [00:30:00] have, I mean, uh, destroy the data. In the right way, you cannot become a data hoarder as well. That’s not, we’re not talking about that, but look for a business.
What are your goals? What are your office style? What is the KPIs that you chase? What successful looks like? Uh, so. If you know what successful looks like for you, what are your KPIs, what are enough to start, you’ll know the minimal data that you have to keep, so, and you can even reduce the price of, say, hey, I will start everything that sometimes could be unmanageable, the amount of data that you, uh, you have, but if you have that data that will be unmanageable.
Drive you to, uh, chase the KPI, to measure the KPIs, to navigate in that ocean, uh, to drive you to the second successful. That’s amazing. But it backs to the point that people have to have clarity about what to do. What is the problem that they [00:31:00] have? What’s the problem that they try to solve? And, uh, what, uh, what is an office of that company?
So if you have it, if you have it, you can, uh, easily, uh, understand the data that you should, uh, start with, you should, uh, keep, uh, for a long time, so on and so forth, it’s, uh, it’s a, it’s a basic, I mean, again, I always like to stress it. Know your North Star. Know what successful looks like for a company.
That’s one problem with a lot of people. Uh, when I say people, I mean, startups try to find the product market fit. They don’t know what is the North Star of the company or what drives that company. Do you just have ideas? Okay, phenomenal. But if you don’t know, uh, what is, uh, your North Star, any wind. will be bad for you and you cannot adjust yourself for the good wind.[00:32:00]
So, uh, that’s the point in the, the data is the same. If you don’t have that basic understanding, probably all you override the data or you delete the data or you start all data and you know, you have some costs for that, for chips that it is, but also it’s not efficient. So, uh, that’s for me is the most crucial, uh, understand what your business, looks like, where do you want to go?
And, when you have that thing extremely clear, you can go down and create a very simple, data strategy. Because another thing that, huge mistake that happens sometimes is people believe that the data strategy should, drive the business strategy. Not at all. No, we’ll have the business strategy and the business strategy will drive the data [00:33:00] strategy.
That’s the importance, to, create that, that vision inside of the companies.
Ryan: career has been pretty much completely working in the U S I mean, I’ve done some work for clients abroad. Um, and that’s been cool, but it’s, it’s much been focused, uh, on the U S and starting and building companies in the U S I know that you have Pretty considerable experience in Brazil. And so I’m curious, right?
Obviously data is data everywhere, but talk to us a little bit about how the business environment and the decision making process and, and, and how folks are using data, how does that differ in the U S and Brazil or more generally abroad?
Antonio: Perfect. Well, when you go to emerging marketing, we don’t have the luxury that you have here in the U. S. that you have Mali. Right? And you have a very good, uh, [00:34:00] startup ecosystem and investor investments, right? Uh, in VCs, private equity, and so on. Uh, emerging marketing, we have, um, scarcity of money. The big challenge for us then, that you cannot make mistake. If you make mistake, it means you die. Your company will die. You want survival. So what I, the big difference for me in emerging marketing like Brazil, we plan more. You have to plan it two or three times more than startups in the U. S. or even in Europe. What’s happening is you have to stress out whole possibilities and say, okay, what’s my plan B, C, and D?
If it does not, uh, work out. If it does not work out. I don’t have a way to raise more money or call to the investor and say, [00:35:00] Hey, yeah, unfortunately we passed it and it does not work out. We need more money to, to do something. Uh, that’s for me, the, the main difference of the two environments here. You can, Because you have more money, also you can take more risks, right?
It’s amazing because also you speed up a bunch of process and you can develop much more things. In emerging marketing, you cannot take that, that same amount of risks because the environment is different. Uh, legally speaking, uh, we don’t have the stability that you have in the US. Uh, you don’t have the financial vehicles that you have in the US.
You don’t have the amount of money, so it’s much more restrict. And, uh, so. You cannot, you cannot use, for example, our fail fast. Uh, so [00:36:00] intense, like we did here in the US, because if you fail, fail fast, amazing, because you can prove, you can pass a lot of things, uh, simultaneously or in very short amount of time to learn and find your product market fit.
And when I go to emerging marketing, uh, you can fail fast. But not that much, right? Because if you fail fast, a lot, you probably, uh, you die. Your company will die. That is the big, uh, the big difference for me. Uh, we don’t have that a lot of space to test things. Uh, What’s a shame is terrible, but it is that reality.
Uh, you have to be more conservative in everything, uh, assumptions and so on and so forth. When you go to the data space. They use data as we use here, but, [00:37:00] uh, we don’t have that, uh, ability to have more space to test space to, to see things. Uh, and even the investor is not that sophisticated like here, right?
Because here also the investors, uh, stimulate you, the good investors, the right money, uh, stimulate you to test, to, to to create an environment that is safe. As long as you don’t do, of course, uh, bizarre things, right? Uh, but the investor, the good investor, the right money, uh, they know that, that, uh, it’s important and they stimulate it.
There is a kind of more complex because even the funds don’t have. So much space, uh, to, you know, uh, to give you that degree of freedom to test and fail, test and fail, and find the product, uh, the product market fit. That’s the, um, the environment. On the other [00:38:00] hand, especially in fintech space, uh, make us in the emerging market be very creative.
You have to be very creative to, to solve, uh, to solve problems, to fundraising, uh, to test things in one way that, uh, you know, you can’t, uh, spend a lot of money. So you can’t, you go and do a survey, for example, uh, You can’t say, okay, how I do it fast and cheap, right? What I see in some startups here, it’s, uh, now it’s a little change, to be honest, because, uh, with the interest rate, not zero anymore, the investors, uh, they now say, Hey, it’s not that, uh, too easy.
But what I’m seeing is, uh, people spend more money without think twice. What [00:39:00] again? Since the interest rate going up and we have, uh, the investors say, Hey, now at least 5 percent of the return, you need to be very conscious about what you are spending, how you are spending, right? It looks like a little more of the behavior that I saw in Brazil.
And another thing, more lean companies, also that’s a behavior that we saw more in Brazil and I’m seeing now that tendency in the U.
Ryan: Yeah. I think, you know, as you talked about it, like I couldn’t help but think to myself like, Hmm, that sounds like a situation where having good data and making better decisions the first time around is a lot more valuable. So I love that. What, what are some of the biggest challenges that you face with data right now?
And how are you looking at them? How are you attacking those problems? Yeah.
Antonio: Data quality. Data quality is the main problem that you have, [00:40:00] uh, right now. Actually you have mainly two, data quality and improve data literacy, right? That, for me, is the two pillars that It’s important. Uh, and, uh, it’s quite, quite difficult when leave without another, of course they can live independent, but, uh, it’s important to that.
The data that you have inside of the company have a good documentation, quality, good shape. You have a reliable source of truth, and so on and so forth. And across the board, in different industries, that point is difficult. Especially when you go to small startups that have to move fast. That’s a big challenge for small startups.
Uh, but even for Big companies. Uh, data quality. That’s issue. And the second issue is, uh, [00:41:00] that will directly also communicate what you are saying about data maturity, right? Uh, the data literacy across the border in different companies is quite low. That is a problem, uh, because, uh, when the people don’t know how to read data or to use data, they just, I don’t know, don’t use.
And, uh, it’s difficult to do what? Take decisions based on data. Or worse, uh, they take decisions, supposed, supposed to be based on data, but as long as they don’t know what they are doing, It’s a garbage, the decision, literally. What I’m seeing sometimes, for example, it’s simple, it could be literally funny, but a lot of people, when you talk about data, say, hey, the correlation in A and B is very high, so I will do something.[00:42:00]
Excuse me. Correlation is not causation, right? So be careful with your decisions. That kind of, uh, theoretically decisions based on data. Let’s imagine that the data is good. You have no problem with data quality. But you have, uh, bad data literacy. The decision like that could drive the company in a very terrible situation.
I always like to joke. It’s not a joke, it’s the truth. with people. And to explain it is, uh, in the summertime, uh, there’s a high correlation of people that, uh, eat ice cream and die drowning. And, uh, if that right correlation, drowning and ice cream, a lot of people can say, it’s easy. Just, uh, stop to, uh, sell ice creams in the summertime and reduce the amount of people that is dying.
Right. But because the correlation is quite high, [00:43:00] it’s not a causation. What cause people die in that case, because they start to go to swim more, exposed to more lakes, ocean, swim pool, et cetera. That’s because it’s hot. That’s the causation. It’s not, uh, the ice cream. It’s the same thing for inside of the company.
A lot of time people see correlations or things like that, but don’t understand what is the causation of that, uh, that situation and take the decision. And fast forward, they look for us in the data team, on the product team, the product data team, and say, well, I think the decision based load data that you sent to me, you sent to me, the correlation was high, and I did something.
Correlation is not causation. Or even the person just read, read some, um, report and take the decisions. So data literacy, it’s extremely, uh, important. And it will become more and more important With [00:44:00] AI, uh, not just, uh, generative AI, predictive AI, data literacy become absolutely something must to have.
Ryan: yeah. I want to give everyone a chance to get to know you. So instead of talking more about data, I want to talk about Antonio. What are, you know, so whether it’s hobbies outside of work or things that you’re interested in, tell us a little bit about you and your background. What do you, what do you like to do?
What makes you happy? What makes you tick?
Antonio: Okay, well, to make me happy, it’s go to the ocean.
Ryan: Yeah, that’s where the swimming example comes from. I love it. Yeah.
Antonio: Go to the ocean. I really like to surf. So, uh, I started to surf, uh, 20 years ago and, uh, I could not stop anymore. Even in the U. S. I tried to find, uh, ways to go to surf. I live in New York, so I go to surf in New York, uh, and I love to travel. to chase waves around the world. So, uh, I [00:45:00] like to go to Indonesia, uh, Hawaii, Australia, Central America, Brazil, uh, always find, uh, good waves to surf.
And, uh, yeah, that’s, uh, that’s make me very happy and relaxed and I can become motivated and find new solutions for my job for things that I’m, uh, I looking for. Uh, yeah. Surf for me is my big, my big, uh, hub and things that, that’s one thing that tells me about myself, the life of the ocean. If I have some free time, I run, I run to the ocean, stay as much as I can, because for me it’s essential.
Ryan: I love it. Yeah. I actually, I grew up at the beach, so I grew up surfing. I have never been very good at it, but I have enjoyed it quite a bit over my life. Um, so if, if you said anything today that anyone was like, wow, I love it. Like I want to reach out. What’s the best way to get in [00:46:00] contact with you?
Antonio: LinkedIn, uh, is the best way to contact with me. I try to answer everybody, add to me the LinkedIn. It’s the best and the fast way to reach me out.
Ryan: Awesome. Antonio, This was honestly an incredible conversation. Like we got to talk about so many separate things, like, you know, products and emerging markets and, you know, data and all of it, it was fantastic. Thank you so much for bringing all of your experience with the entrepreneurial and international community.
It, for me, was kind of jam packed with new things that I think the listeners will get a lot out of. So thank you so much for coming on.
Antonio: Thank you for having me. It was a pleasure to talk with you and with your audience.
Ryan: I also, speaking of them, want to thank the audience. If you made it this far, thanks for listening to the whole thing. Please make sure to, you know, like, and subscribe and tell a friend and give us a good review. Uh, all of that stuff really helps to keep the podcast going. Antonio, thank you so much again.
And this has [00:47:00] been another episode of the Making Better Decisions podcast. Thanks for listening.
Outro: That’s a wrap for today’s episode of making better decisions for show notes and more visit, making better decisions dot live a special thank you to our sponsor canopy analytic canopy. Analytic is a boutique consultancy focused on business intelligence and data engineering. They help companies make better decisions using data for more information, visit canopy analytic.
com. There’s a better way. Let’s find it together and make better decisions. Thank you so much for listening. We’ll catch you next week.
Sign up for our newsletter
Stay up to date with the roadmap progress, announcements and exclusive discounts feel free to sign up with your email.